Forty-three percent of the 521 artificial intelligence (AI) health devices authorized by the US Food and Drug Administration (FDA) between 2016 and 2022 lack publicly available clinical validation showing they were tested using real patient data, a new study found.
The study, from the University of North Carolina (UNC) at Chapel Hill, highlights an urgent need for more public data and clearer standards around AI medical devices, the researchers say. And they're calling for clinicians to get involved.
"The number of devices that we found clinical validation data on — a lot of people think, oh, that's the big news," said lead author Sammy Chouffani El Fassi, an MD candidate at the UNC School of Medicine and research scholar at Duke Heart Center. "But [that's] not as [big] as realizing that there's no standard yet."
That is, there's no standard for clarifying "which devices work really well versus which could work well with more [validation]," Chouffani El Fassi said.
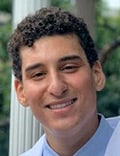
It's important to note that just because data is not publicly available doesn't necessarily mean it doesn't exist.
The researchers based their analysis on brief, public-facing summaries. But regulators consider "thousands, if not tens of thousands of pages" of information about each tool, according to Troy Tazbaz, director of the FDA's Center for Devices and Radiological Health, Digital Health Center of Excellence. Much of that information is confidential, and it can include real patient data.
Still, the uncertainty could make clinicians more hesitant to adopt new tools, said Chouffani El Fassi. "Physicians probably won't trust a device if it wasn't exposed to confounding variables in real-world implementation," he said.
More than that, the study poses an opportunity for clinicians and academic institutions to participate, Chouffani El Fassi said. "It would be great if more researchers out there were testing devices that are already on the market and seeing how they work in patients."
Nearly all of the 521 tools were Class II devices, which are considered to be of moderate risk to patients. These tools are typically authorized by the FDA based on their similarity to existing technology that "would have had clinical validation the first time it got approved," said Nigam H. Shah, PhD, chief data scientist for Stanford Health Care who was not involved in the study.
Of the 43.4% of tools lacking available clinical validation, radiology devices accounted for more than half. Most of those devices aid image archiving and communication, functions that may not need to be prospectively validated, according to Shah.
"You can test [image archive tools] on data of cats and dogs and cars," he said.
How Clinicians Can Help
The more striking finding, Shah said, is the rapid spike in AI medical device authorizations, which skyrocketed from an average of two per year in 2016 to 69 per year in 2022. (The full list has since grown to 950 authorized AI and machine learning-enabled devices.)
"We have gone nuts in terms of the number of devices that are being put up for approval or authorization," he said. While that's a huge workload increase for FDA regulators, he added, "academics can step up."
Clinicians could validate one AI device as part of their fellowship, for instance, or device companies could approach public-private groups, like the Coalition for Health AI, to carry out prospective validation.
That research can be "very simple," said Chouffani El Fassi. "You don't necessarily need some big, crazy study."
Consider an AI tool that reads CT scans, potentially flagging strokes and hemorrhages of the brain before a human radiologist has time to review the scans.
Radiologists could help clinically validate the tool by comparing the time to diagnosis with and without the use of AI, or by rating its usefulness on a five-point Likert scale.
"The Likert scale can be somewhat subjective," Chouffani El Fassi said. "But if the physicians feel like the product is wasting their time, not easy to use, or not helpful to their practice when used on real patients, then you have an understanding of clinical value."
That information could boost adoption of AI devices, said Shah.
Just 38% of physicians surveyed by the American Medical Association last year said they were using AI, despite 65% considering AI advantageous to healthcare.
Many clinicians are hesitant to use new tools because the benefit to their practice may not justify the cost. FDA-authorized devices "might work, but does their use lead to better care? We have not answered that question," Shah said.
Need for Consistent Language
The UNC researchers' analysis also revealed a lack of consistent language for different methods of evaluating AI health tools.
The FDA, academic researchers, device developers, and manufacturers may use different terms for prospective, retrospective, and clinical validation. Because those methods result in different quality evidence, the researchers argue that the FDA should include clear distinctions in its authorization summaries.
To that end, the researchers created a set of straightforward definitions for each method. This new "clinical validation standard" could guide different stakeholders as they test devices and inform potential users about technologies.
Most public-facing summaries about device authorization are written by manufacturers, not the FDA, said Tazbaz.
Standardized language could help "better categorize the level of clinical validation that a tool has," Chouffani El Fassi said.
"There's really a lot of opportunity to improve the field, and for people to be pioneers in improving this technology, and then using it to improve people's lives," he said.
https://www.medscape.com/viewarticle/nearly-half-ai-health-tools-fall-short-public-data-2024a1000ftu
No comments:
Post a Comment
Note: Only a member of this blog may post a comment.